According to a recently released MarketWatch analysis(1), the global Motion Control Encoders market is predicted to grow at an annual rate of 4.6%, exceeding $2.1 billion by 2026. Industries like chemical manufacturing, industrial automation, aerospace, and consumer electronics will all be impacted by this growth. Some of the key motion control manufacturers mentioned in this analysis include
- Renishaw
- Dynapar
- BEI Sensors
- Baumer Group
- Hengstler
- Broadcom
- Tokyo Sokuteikizai
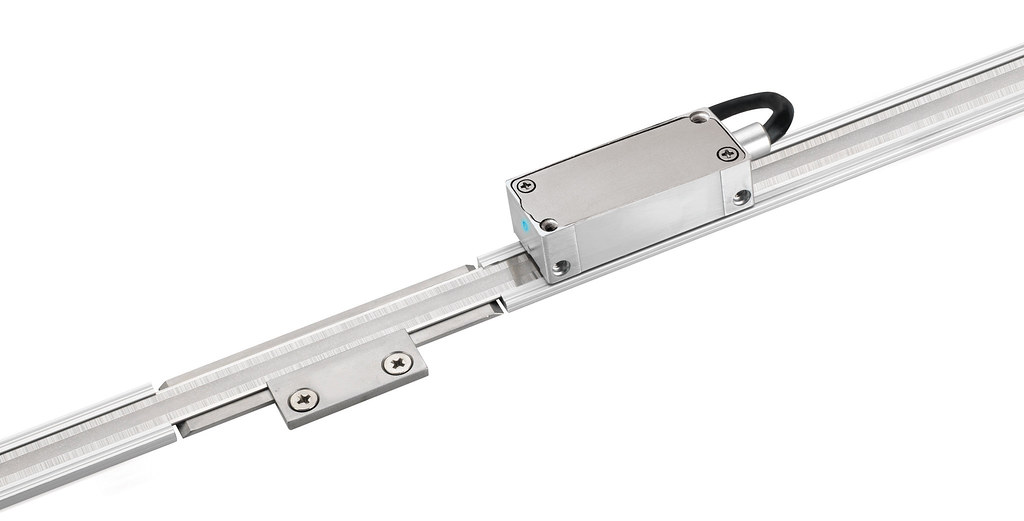
Robotics, automation, sorting, imaging, and plotting applications use encoders. The sensing devices provide feedback to determine direction, position, count, or speed. Absolute and incremental encoders can both achieve these ends, but operate differently using different methods of implementation.
In a nutshell, an absolute encoder provides unique position values based upon a digital value of an object’s angular position or motion. These values occur between a stationary pickup device (a zero point) and a moving encoding device, for example, a slotted disc on the shaft of a rotary encoder.
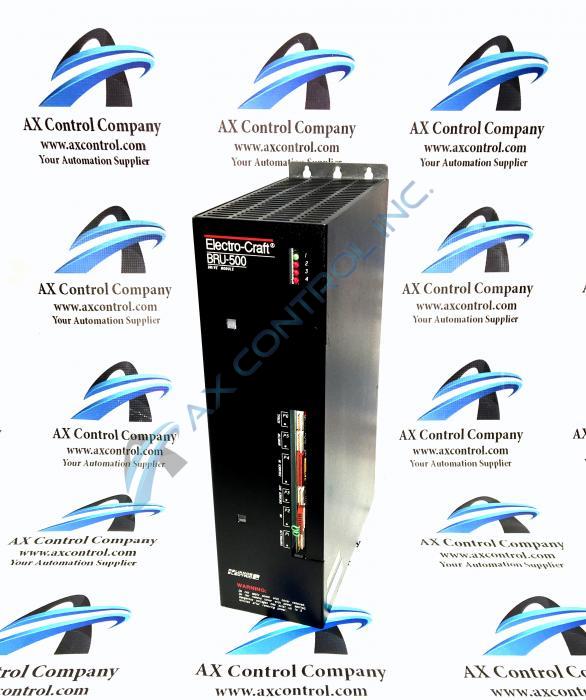
Incremental encoders, on the other hand, offer an output signal based upon two square waves that each correspond to a unit of travel read by a photodiode as the load moves. Because incremental encoders begin their count at zero at startup or during a power disruption, they offer no safeguards or reference point regarding position before these events.
Rotary vs Linear Encoders
It’s important to realize the terms “absolute” and “incremental” refer to movement identification. The terms “rotary” and “linear” are more about geometries. It is important to recognize rotary and linear encoders may be either absolute or incremental.
Linear
A linear encoder, as the name implies, measures motion along a line or path. It is designed with a coded strip, or scale, and a sensing head. Incremental linear encoders zero out after any kind of reset. For example, a loss of power or power-down will cause a reset. On the other hand, absolute linear encoders offer their exact position by creating a unique position signal. This position signal is converted into a digital or analog signal for readout purposes. But remember that linear encoders may be optical or magnetic.
For a long time, optical linear encoders were the only option for resolutions below five microns. They offer high resolution and accuracy and can be used in areas with high magnetic fields.
However, optical encoders are mechanically fragile due to the glass optical disk. This can shatter due to impact or vibration. Shock and vibration can also negatively affect internal positioning.
Magnetic linear encoders are less affected by shock and vibration. However, these units use magnetic reader heads combined with a magnetic scale to determine position. This feature creates a sensitivity to anything interfering with the magnetic field such as iron, steel, or nearby magnetic fields.
Rotary
Rotary encoders are used to track rotational motion, including the shaft movement of a number of industrial and commercial devices. Incremental rotary encoders tend to be less complex and more cost-effective than similar absolute encoders, and are adequate for simple pulse counting or for frequency monitoring applications where the resolution is no more than 50,000 PPR. Absolute rotary encoders offer a higher resolution of up to 65,536 pulses per revolution. These encoders also offer multiple interface options including parallel, serial, Ethernet, Fieldbus, and analog.
(1) https://www.industryresearch.co/global-motion-control-encoders-market-15934813
You must be logged in to post a comment.